AI Large Language Models and Supervised Fine Tuning
Security Analyst, Malware Researcher, New Technology Researcher Evangelist

This blog post is aimed at the intermediate level learner in the fields of data science and artificial intelligence. If you would like to read up on some fundamentals, here is a list of useful resources.
Large Language Models
Generative large language models (LLMs) based on the transformer architecture have become a very popular technology in natural language processing (NLP). As most readers are aware, there are many popular public LLMs out there which are used daily via a web-based or JSON restful API interface.
Some examples are:
- ChatGPT (OpenAI)
- Gemini (Google)
- Claude (Anthropic)
- Llama (Meta)
For these very large models, the vendors have built enormous compute resources with many hundreds of Tensor or Graphics Processing Units (TPU/GPU) to train them. Clearly, resources like these are out of reach for the typical information security researcher and/or data scientist.
You can think of these large models as being the digital brain equivalent of a very bright high schooler—or even University graduate today—and they are truly a wonderful resource. Like many University graduates, these models have a broad array of generalized knowledge, but they are typically not “expert” in any specific domain of knowledge.
As an analogy, when we hire a young employee in any specific industry, the new employee’s initial tasks are to get trained on the industry domain focused knowledge, and of course our own local process and procedures.
In the data scientist community, there is a commonly used resource called “Hugging Face” to which some of the very large public models have chosen to be published openly. Thus, the obvious question becomes, can I take one of these large models and send it to school? Can I further train a large model in a very domain specific knowledge or task area so that this model becomes a “professional” or very much more capable in my chosen knowledge domain. The answer is “YES YOU CAN,” and furthermore, you can do this with limited GPU resources.

Supervised Fine Tuning
The process we use to send a large language model to be schooled in a domain specific knowledge realm is called Supervised Fine Tuning (SFT).
Supervised Fine Tuning of an LLM involves:
- Adjusting the weights and parameters of an existing pre-trained model to improve its performance in a specific knowledge domain
- Training of the model on new data that is specific to the task or domain knowledge of interest which updates the model’s weights to adapt to the new data
Aside from knowledge domain adaptation, why would we want to perform Supervised Fine Tuning on a model?
- Better alignment with desired behavior, and the lowering of irrelevant or undesirable results from the generalized model.
- To customize the resulting model for a specific business workflow.
- To improve the efficiency of the model in a limited TPU/GPU resource environment.
- To avoid a known issue called “catastrophic forgetting,” otherwise said to help retain general capabilities and adapt/align more closely with the new task at hand.
If all this sounds a lot like you are re-training a human to be more proficient in a specific chosen profession, your interpretation is 100% correct. This is the AI-LLM equivalent of the same process. Supervised fine tuning is quite literally tweaking the digital brain to align with a desired new profession!
When performing SFT, we have choices to make. We can either take the approach of:
- Full Fine Tuning whereby we adjust all of the weights of the model. This is a comprehensive approach but is also resource intensive and not well suited to a limited TPU/GPU environment.
- Transfer Learning / Repurposing whereby we take an existing model, freeze most but not all its neural network layers, and then fine tune a subset of those layers to achieve the desired resource. Conveniently for us, this is a much less resource intensive task and can often be achieved in a single GPU limited resource environment.
As you might suspect, there have been some algorithmic efficiencies invented to help us with the approach of transfer learning. In particular, Parameter Efficient Fine Tuning (PEFT) is an approach that only adjusts a small subset of model parameters while the majority of the pre-trained parameters remain frozen. This is a very important approach, and the vendors that have openly published LLMs are trying to facilitate the use of their models by publishing different parameter sized models anticipating the SFT process will be used.
There is a notable Python package which is focused on the challenges of a single GPU environment called “Unsloth” published by https://unsloth.ai. Unsloth does a great job of getting you up and running for SFT and I would recommend investigating it.
Meta’s LLAMA 3.1 model for example is published in three parameter sizes. For the purposes of SFT, the different parameter sized models listed below are re-published in a quantized form as a part of the Unsloth framework effort.
- 8 billion parameters
- SFT can occur on a single consumer grade GPU with 24GB video ram (VRAM).
- 70 billion parameters
- SFT would need more VRAM, typically around 40GB but can still be performed on a single GPU.
- 405 billion parameters
- SFT will require a larger multiple GPU architecture.
To maximize efficiency, some additional techniques have been developed to improve the process in a limited resource environment.
- LoRA stands for low rank adaptation which is a 16-bit word length method designed to reduce memory requirements. Rather than adjust all model parameters, LoRA injects trainable low-rank matrices into specific layers of the model to be adjusted.
- QLoRA is quantized LoRA, and improves further on the LoRA method by using a 4-bit word length to further lower video RAM requirements. QLoRA represents a trade-off between re-training time and memory resource usage and has become very popular in single GPU environments.
Data Preparation
To be dead honest for a moment, as I first approached re-training the LLAMA3.1 LLM published by Meta, I had this sort of reverence for the technology. Although I was well aware of data science fundamentals, the hype cycle of the capabilities surrounding LLMs was in full swing, and I honestly thought these things could do nearly anything.
With that in mind, my first attempt at SFT was to grab large chunks of textual data on the knowledge domain of choice, and just literally throw it at the wall, so to speak. Did anything stick? Absolutely NOT… in fact, my result was a very confused digital brain that stuttered, repeated itself, converged on single sentence answers, and was, frankly, mentally disabled.
Of course, failure is a fabulous learning opportunity, so I went back to the drawing board and learned that the best path towards fine tuning a generative LLM is in fact to adopt the goal of producing an “instruct model” which requires you to prepare the training data in a “question / answer” pair format.

Naturally, my next reflexive response was to groan aloud. I have several hundred megabytes of data that I needed to weed through and prepare in “question/answer” pairs? Whiskey Tango Foxtrot!
In fact, the requirements for a high-quality result are even more stringent if we want to do this right. We need to ensure that:
- Source data all has task specific or knowledge domain relevance.
- The data is high in quality and preferably in quantity also.
- All HTML links, and non-relevant information, and perhaps even some metadata will need to be cleaned up, perhaps corrected, or removed.
In other words, welcome to the drudge work of “data science” which is not in the least attractive and no one really talks about.
In conjunction with these, I was involved in a tremendous amount of reading and research in the field of Artificial Intelligence, and one night around 3am, I had an epiphany which really ended up being more a facepalm moment when it came to me. Why not use another LLM to prepare my data?

It so happens that OpenAI’s “chat-gpt-mini-4o” model is extremely proficient at processing textual data. All I needed to do was construct intelligent prompt engineering for the data I was going to feed into OpenAI’s LLM to get my data prepared in a better form.
After working on several different attempts, I also discovered some external helpful resources. A portion of this prompt engineering was inspired by the “LLM Engineers Handbook” (Authors: Paul Lusztin, and Maxime Labonne) which can be found here at https://www.google.com/books/edition/LLM_Engineer_s_Handbook/jHEqEQAAQBAJ

In conjunction with this prompt engineering, I wrote a Python script to interact with OpenAI’s API to present the data. While doing this, I set the “temperature” parameter for the API queries to 0.7 to yield greater creativity in responses, and I also requested the API returned sixteen different responses for each single data item presented as a query.
More specifically, the OpenAI API’s temperature parameter affects the computation of token probabilities when generating output from the large language model. The temperature value ranges from 0 to 2, with lower values indicating greater determinism and higher values indicating more randomness.
Further, because I am a proficient Python programmer, I wrote the code as a multi-threaded script and tuned it such that I would just barely stay below the OpenAI upper token throttling threshold.
Once your data has been properly prepared, you can choose to upload it to a Hugging Face account, if you would like. This makes it easier to integrate with SFT Python scripts or Jupyter notebooks. Importantly, don’t forget to set your prepared data to “private” on Hugging Face if you do not want to publish openly.
Below is a screenshot of some Jupyter notebook portions of an SFT script so that you can see how you might go about performing SFT using Llama3.1 and the 8 billion parameter model.
In this example, we are using an Information Security domain knowledge dataset called “White Rabbit” to perform SFT on the Llama3.1 model. I did not have to perform any data preparation steps on the White Rabbit dataset as that work had already been done for me.
These script snippets are functional in an Nvidia RTX4090 GPU, 24GB VRAM environment. Note that these are just some fundamental screenshots, not the complete notebook, designed only to give you a sense of what is required.





In conclusion, the above information covers the process of supervised fine tuning and re-training an LLM for domain specific knowledge purposes. Once retrained, you have the option of publishing your new model on Hugging Face and, of course, using your new model for your own inference query purposes.
Happy AI-LLM hunting! If you want to know more, you can attend the Antisyphon class titled “AI for Cyber Security Professionals” by Joff Thyer and Derek Banks. If you are interested in security assessment of AI models, please contact Black Hills Information Security contact form at this URL: https://www.blackhillsinfosec.com/contact-us/
Fascinated? You can learn more straight from Joff himself:
Introduction to AI for Cybersecurity Professionals
Available live and on-demand!
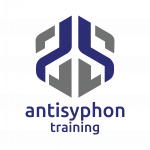